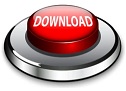
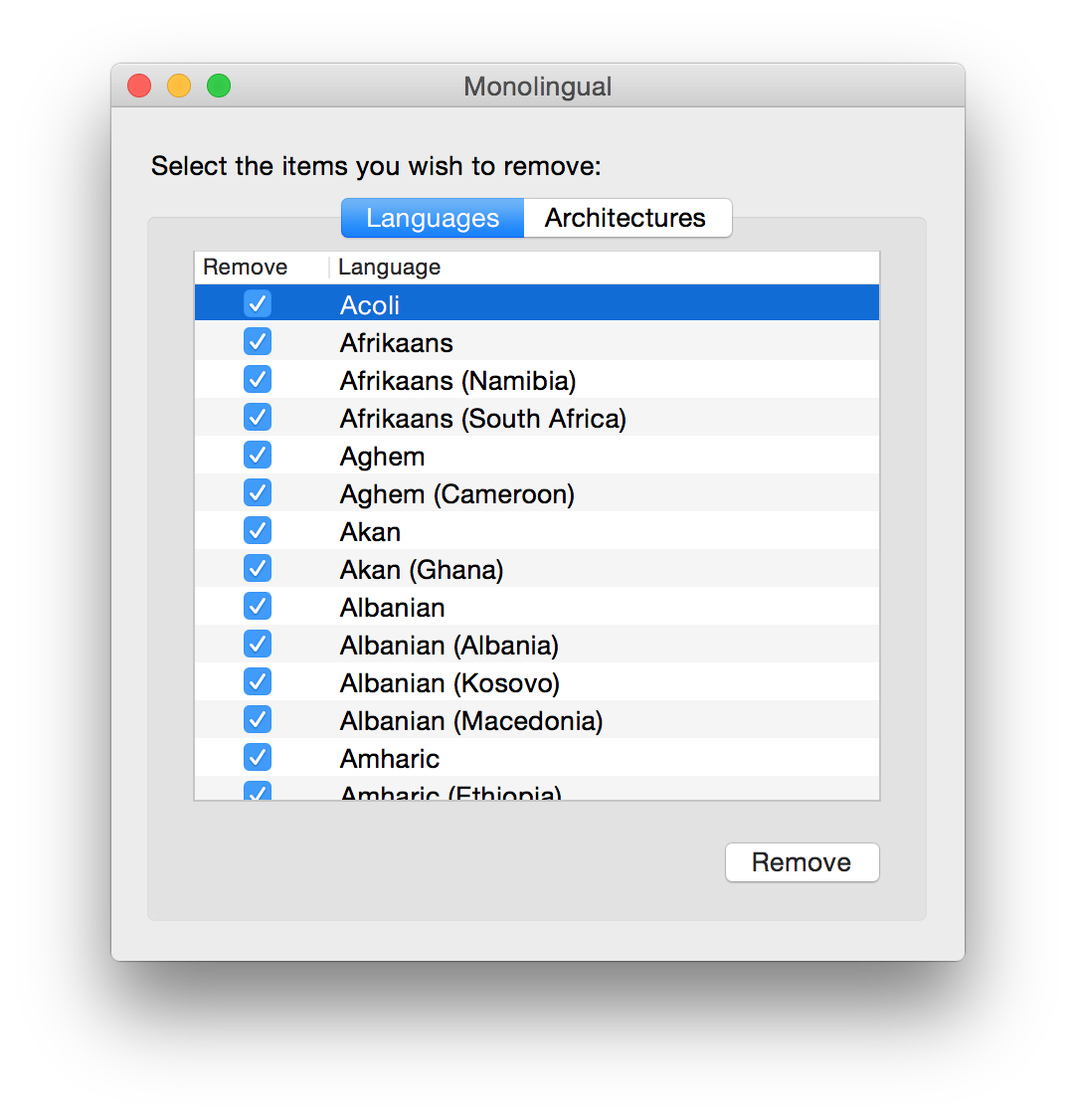
The tokens ”The” and ”car” are used to extract the two kinds of embeddings that are merged before being used as input for the encoder RNN Finally, in Section 6 we presents our conclusions and the future work.įigure 1: Merging external embeddings with the normal NMT embeddings in the encoder side. In Section 4, we introduce the experimental setup and show our results, while in Section 5 we discuss our solution. Then, we introduce our modification to enable the use of external word embeddings. In the following section we briefly describe the state-of-the-art NMT architecture. Our results show that this method seems effective in a small training data setting, while it does not seem to help under large training data conditions.
MONOLINGUAL BIG SUR HOW TO
We show that the network is capable to learn how to translate from the input embeddings while replacing the source embedding layer with a much smaller feed-forward layer. Because of the softmax layer, the same idea cannot be applied straightforwardly to the target side, hence we continue to use sub-words there. This should be true particularly for the low-resource settings, where parameter transfer has shown to be an effective approach (Zoph et al., 2016). The intuition is that the network should learn to use the provided representations, which should be possibly more reliable for the rare words. We do it by integrating the usual word indexes with word embeddings that have been pre-trained on huge monolingual data. In this paper, we propose to keep the source input at a word level while alleviating the problem of rare and OOV words. The drawback of this approach is that it generates longer input sequences, thus exacerbates the handling of long-term dependencies (Bentivogli et al., 2016). The solution up to this moment is to segment words into sub-words (Sennrich et al., 2015b Wu et al., 2016) in order to have a better representation of rare and OOV words, as parts of their representation will be ideally shared with other words. In fact, being NMT a statistical approach, it cannot learn meaningful representations for rare words and no representation at all for OOV words. Hence, this approach does not allow to leverage huge quantities of monolingual data.ĢOne consequence of the scarcity of parallel data is the occurrence of out-of-vocabulary (OOV) and rare words. Unfortunately, this method introduces noise and seems really effective only when the synthetic parallel sentences are only a fraction of the true ones. This consists in enriching the training data with synthetic translations produced with a reverse MT system (Bertoldi and Federico, 2009). Recently, back-translation (Sennrich et al., 2015a) has been proposed to leverage target language data. NMT mainly relies on parallel data, which are expensive to produce as they involve human translation. Sfruttando word embedding appresi da testi inglesi estratti dal Web, siamo riusciti a migliorare un sistema NMT basato a parole e addestrato su 200.000 coppie di frasi fino a 4 punti BLEU.ġThe latest developments of machine translation have been led by the neural approach (Sutskever et al., 2014 Bahdanau et al., 2014), a deep-learning based technique that has shown to outperform the previous methods in all the recent evaluation campaigns (Bojar et al., 2016 Cettolo et al., 2016). I nostri risultati preliminari mostrano che i nostri metodi, pur non sembrando efficaci sotto condizioni di addestramento con molti dati (WMT En-De), risultano invece promettenti per scenari di addestramento con poche risorse (IWSLT En-Fr). In questo lavoro presentiamo dei metodi per fornire ad una rete word embedding esterni addestrati su testi nella lingua sorgente, consentendo quindi un vocabolario virtualmente illimitato sulla lingua di input.
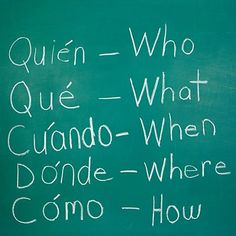
La soluzione più adottata consiste nel segmentare le parole in sottoparole, in modo da consentire rappresentazioni condivise per parole poco frequenti. Una limitazione della NMT è comunque la difficoltà di apprendere rappresentazioni di parole poco frequenti. La traduzione automatica con reti neurali (neural machine translation, NMT) ha ridefinito recentemente lo stato dell’arte nella traduzione automatica introducendo un’architettura di deep learning che può essere addestrata interamente, dall’input all’output.
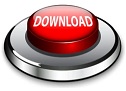